Large language models (LLM) are a type of well-trained AI on extensive amounts of text data. These LLMs are excellent at generating text, translating languages, creating content in different genres, and answering questions.
Open-source LLMs are freely available for everyone to use and modify. This makes the LLMs accessible for a wide range of applications. These models have become important tools for businesses and individual users as they offer solutions for various tasks.
In 2024, the scope of LLMs will continue to expand with new models offering even more capabilities and improvements. There’s an LLM available suited to your needs whether you’re a developer, writer, or researcher.
What are Open-Source Large Language Models?
Open-source LLMs are trained on large text datasets to generate human-like language. Their source code is freely available, allowing anyone to use, modify, and share them. This open access encourages global collaboration, as developers can improve and add new features.
Organizations save time and resources by using these models. Moreover, these LLM models are versatile and excel in various NLP tasks. They also promote transparency and responsible AI practices.
How Do Large Language Models Work?
Large language models are equipped with large amounts of text-based data. This textual data is derived from the internet, published articles, and books. LLMs understand the connection between words and predict results based on the pattern.
LLM uses learning techniques to analyze information and provide results. LLMs can do the various actions given the appropriate training, like:
- Address the consumer inquiries
- Summarize email discussions
- List action items from the meeting notes
- Generate blog post outlines
How Do LLMs Learn Linguistic Context?
Large language models can understand whether a user means an animal or an object when they type “bat.” The models predict what the user will type next and respond based on their training data. It’s important to consider the parameters when talking about an LLM’s training data.
Parameters are the variables used to train the model. The more parameters an LLM has, the better it can understand and generate complex text. Parameters help the model grasp the nuances of language. This makes it more effective in various applications from generating creative content to providing accurate responses.
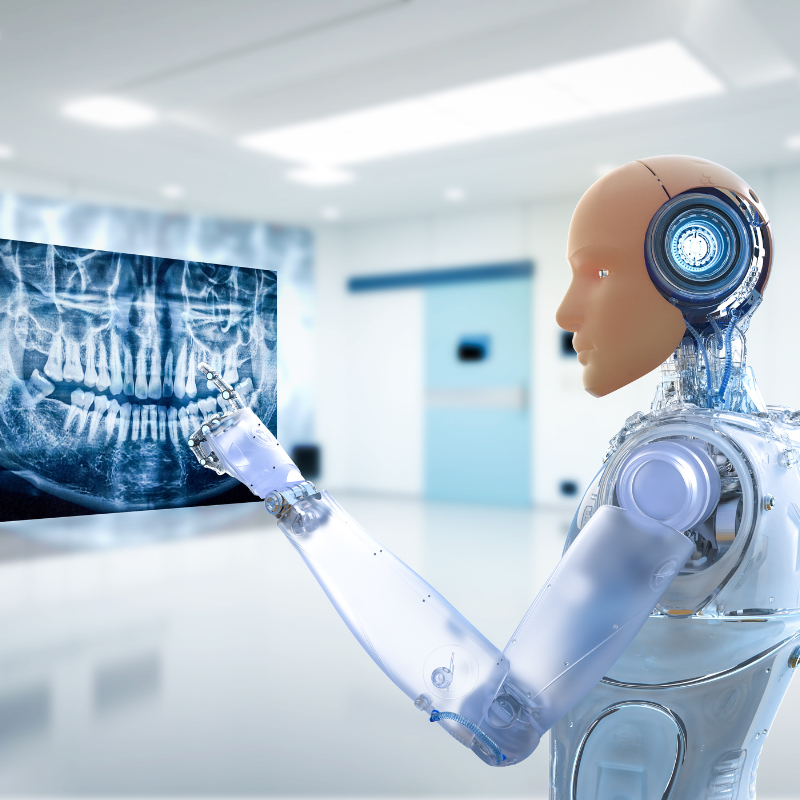
10 Best Large Language Models for 2024
Today, there are many large language models available for marketing. Here we’ve compiled a list of the most popular ones and provided key details to help you choose the best fit for your needs.
Grok AI
Grok AI helps in text summarization and comprehension using advanced NLP algorithms. It quickly extracts key insights from complex documents with deep learning. It helps researchers, businesses, and content creators available only on Twitter. Legal professionals use it for document summarization. Educators and students benefit from efficient learning and real-time insights.
LLaMA 3
LLaMA 3 is developed by Meta AI which supports 8,000 tokens for complex input handling. It comes in sizes up to 65 billion parameters. LLaMA 3 performs well in tasks like question answering and sentiment analysis. Its scalability makes it efficient for large datasets and modern projects.
BERT
BERT captures bidirectional context for better language understanding. BERT is created by Google for text categorization, question answering, and named entity recognition. BERT enhances recommendation engines, chatbots, and search engines. Its ability to grasp nuances improves natural language processing.
BLOOM
BLOOM focuses on creating logical, contextually accurate language. It is developed by the Allen Institute for AI. This LLM uses transformer-based architectures for generating fluent responses. BLOOM is employed in document classification, dialogue production, and text summarization. It automates content generation and enhances chatbot conversations.
Falcon 180B
Falcon 180B is designed for efficient language processing with high-speed capabilities. It’s ideal for real-time applications like question answering and text completion. Businesses leverage it for social media research and chatbot development. Falcon 180B helps in quick and accurate text processing.
XLNet
XLNet uses a permutation-based pre-training method to improve language understanding. It comprehends long-range dependencies and relationships in text. XLNet is used for text creation, question answering, and language modeling. It generates contextually relevant and coherent text.
OPT-175B
OPT-175B optimizes speed and performance for large-scale text data. The LLM is built on a transformer architecture for accurate language generation. OPT-175B is used in document categorization, sentiment analysis, and text summarization. Its optimization allows for efficient and rapid text data processing.
XGen-7B
XGen-7B specializes in generating creative and complex content. It produces varied and engaging prose for marketing and storytelling. XGen-7B understands complex linguistic patterns and nuances. It is used in dialogue systems, creative writing, and other content creation tasks.
GPT-NeoX and GPT-J
GPT-NeoX and GPT-J are efficient and scalable for diverse NLP tasks. These LLMs help in language understanding, text completion, and chatbots. These models are versatile for tasks like sentiment analysis and code generation. They are valuable tools for advanced language processing needs.
Vicuna 13-B
Vicuna 13-B is built for scalable and efficient language processing. It handles large text datasets using transformer technologies. Vicuna 13-B is utilized in question answering, text summarization, and language modeling. It’s suitable for sentiment analysis and content recommendation systems.
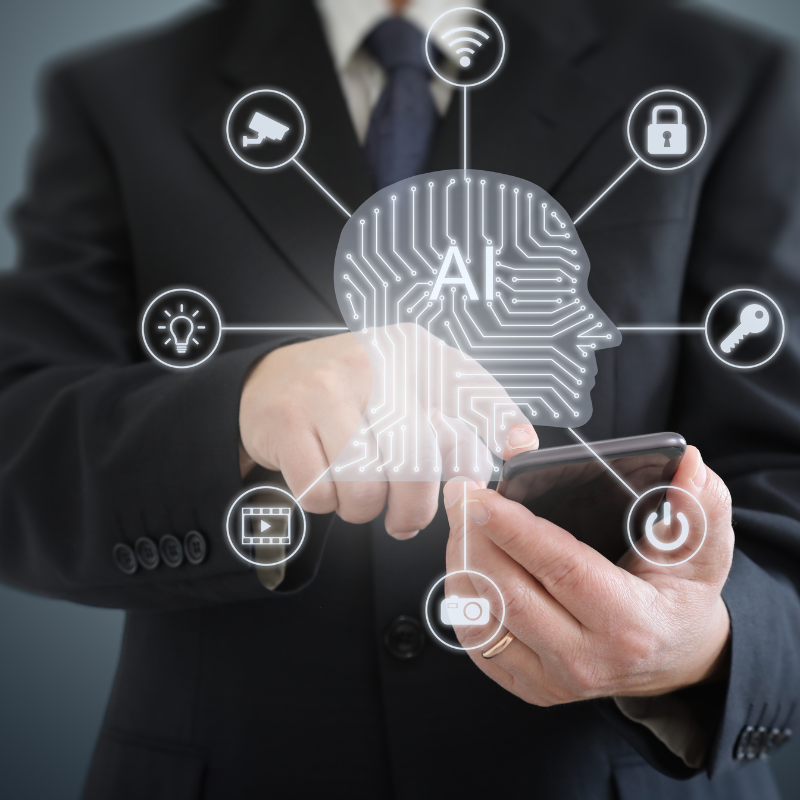
How Will You Choose The Right LLM?
Selecting the best Large Language Model involves considering various factors. Here are the factors to help you find the best fit:
- Task Requirements: Firstly, you need to identify your NLP task, like text summarization, sentiment analysis, or question answering. All of the above LLMs help in different areas, such as BERT for sentiment analysis and Grok AI for text generation.
- Model Capabilities: You should consider the strength and features of each model, like BERT’s bidirectional context understanding or XLNet’s long-range dependency modeling.
- Size of the Dataset: Smaller models like LLaMA 2 might be better for limited datasets whereas larger models require more data and resources.
- Computational Resources: Bigger models like Falcon 180B need substantial computational power. You have to ensure your infrastructure can support the model’s size and complexity.
- Performance Metrics: Review benchmark results for models like BERT and GPT series to assess their effectiveness.
- Experimentation and Evaluation: You can test several models to find the best fit for your use case, evaluating metrics like accuracy and precision.
Closing Thoughts
In 2024, Large Language Models (LLMs) will dominate Natural Language Processing (NLP) with their advanced text generation capabilities. Open-source models like BERT, Grok AI, and XLNet offer affordable solutions, democratizing AI technology. The right choice depends on task needs, model features, and computational resources.